Juniorprof. Britta Velten Biological Data Science
Our group works at the interface of data science, machine learning and the life sciences with the aim to develop the computational tools and statistical methods that are required to translate large-scale molecular data sets (‘omics data’) into biological insights and novel discoveries.
Complex experimental designs and novel experimental technologies enable researchers to study tissues, organisms and biological processes at unprecedented resolution and scale. This produces a wealth of diverse molecular data ranging from transcriptomics and proteomics to epigenome profiling and metabolomics. Jointly this data can shed light on the molecular heterogeneity and regulatory mechanisms of biological processes. For this, data integration across different technologies, model systems, scales and environments is crucial to leverage such data for biological discovery.
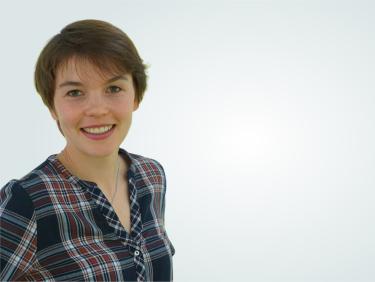
Our research
Our group combines probabilistic machine learning and statistical reasoning to enable data integration, explorative data analysis, robust statistical inference and meaningful visualization for modern omics data. Our focus lies on the development of latent variable models for data integration and dimension reduction, the design of computational approaches for modelling temporal and spatial omics data and the use of causal inference to uncover regulatory mechanisms from molecular measurements. The tools and methods we develop are open-source and available from GitHub, Bioconductor and PyPI.
Integrative data analysis
Modern technologies enable researchers to study biological organisms and processes simultaneously on different molecular layers and in diverse biological contexts. To enable a joint analysis of the resulting data, we develop methods for the supervised and unsupervised integration of multi-modal omics data. Our methods facilitate the joint analysis of data arising from multiple omics technologies and different biological contexts (e.g. species, environments, individuals, tissues or cell types) in a data-driven manner. Thereby, we can identify the major sources of biological variation from high-dimensional complex data sets and pinpoint differences and commonalities across molecular layers or biological contexts. In collaborations we make use of such methods to characterise biological heterogeneity, identify core molecular processes underlying development and pinpoint their molecular drivers. For example, this enabled us to identify central axes of disease heterogeneity in blood cancer or to dissect the interplay of epigenetic and transcriptomic changes during cell fate commitment and lineage formation.

Spatio-temporal modelling
With advances in high-throughput omics technologies, time- and space-resolved molecular measurements at scale are increasingly feasible. The resulting molecular read-outs with temporal and spatial resolution offer new opportunities to study the dynamic and contextual properties of a biological system and can thereby uncover novel traits that would not be visible without the temporal or spatial context. To extract such insights from the data, we develop methods for the identification of temporal and spatial patterns from large-scale omics data sets with temporal and spatial resolution. These methods can provide direct insights into the molecular drivers of temporal dynamics and spatial organization and enable the direct comparison across different biological contexts (e.g. species, environments, individuals, tissues or cell types). Applications of such methods include modelling developmental gene expression programs, microbiome dynamics, spatial patterning of expression at the cellular and subcellular level or transcriptomic and epigenetic dynamics on the single cell level during development.

Regulatory mechanisms & causal inference
The ability to combine molecular read-outs with targeted or non-targeted interventions opens up new opportunities to gain insights into the regulatory molecular mechanisms and genetic dependencies of organismal development and plasticity. In our research, we apply causal modelling and statistical invariance principles in order to reveal common principles across model systems and environments and pinpoint causal mechanisms on the molecular level. For this, we develop methods for the analysis of CRISPR-based intervention studies with molecular read-outs to dissect gene regulatory mechanisms and we design models for the identification of universal mechanisms by integrating data across different environmental contexts, species or chemical and physical perturbations.
